Abstract:
Covariate adjustment can improve precision in analyzing randomized experiments. With fully observed data, regression adjustment and inverse probability weighting are asymptotically equivalent in improving efficiency over unadjusted analysis. When some outcomes are missing, we consider combining these two adjustment methods with inverse probability of observation weighting for handling missing outcomes, and show that the equivalence between the two methods breaks down. Regression adjustment no longer ensures efficiency gain over unadjusted analysis unless the true outcome model is linear in covariates or the outcomes are missing completely at random. Propensity score weighting, in contrast, still guarantees efficiency over unadjusted analysis, and including more covariates in adjustment never harms asymptotic efficiency. Moreover, we establish the value of using partially observed covariates to secure additional efficiency by the missingness indicator method, which imputes all missing covariates by zero and uses the union of the completed covariates and corresponding missingness indicators as the new, fully observed covariates. Based on these findings, we recommend using regression adjustment in combination with the missingness indicator method if the linear outcome model or missing complete at random assumption is plausible and using propensity score weighting with the missingness indicator method otherwise. This is a joint work with Anqi Zhao and Peng Ding.
About the Speaker:
Fan Li is a professor in the Department of Statistical Science, with a secondary appointment at Biostatistics and Bioinformatics at Duke University. Her primary research interest is statistical methods for causal inference, with applications to health and social sciences. She also works on Bayesian analysis and missing data. She is the editor for Social Science, Biostatistics and Policy of the Annals of Applied Statistics, and an elected fellow of the American Statistical Association and the Institute of Mathematical Statistics (IMS).
Your participation is warmly welcomed!
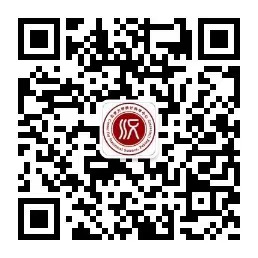
欢迎扫码关注北大统计科学中心公众号,了解更多讲座信息!