报告人: Yingjie Feng(Tsinghua University)
时间:2023-09-14 15:10-17:00
地点:Room 216, Guanghua Building 2
Abstract:
Dyadic data is often encountered when quantities of interest are associated with the edges of a network. As such it plays an important role in statistics, econometrics and many other data science disciplines. We consider the problem of uniformly estimating a dyadic Lebesgue density function, focusing on nonparametric kernel-based estimators taking the form of dyadic empirical processes. Our main contributions include the minimax-optimal uniform convergence rate of the dyadic kernel density estimator, along with strong approximation results for the associated standardized and Studentized t-processes. A consistent variance estimator enables the construction of valid and feasible uniform confidence bands for the unknown density function. We showcase the broad applicability of our results by developing novel counterfactual density estimation and inference methodology for dyadic data, which can be used for causal inference and program evaluation. A crucial feature of dyadic distributions is that they may be “degenerate” at certain points in the support of the data, a property making our analysis somewhat delicate. Nonetheless our methods for uniform inference remain robust to the potential presence of such points. For implementation purposes, we discuss inference procedures based on positive semi-definite covariance estimators, mean squared error optimal bandwidth selectors and robust bias correction techniques. We illustrate the empirical finite-sample performance of our methods both in simulations and with real-world trade data, for which we make comparisons between observed and counterfactual trade distributions in different years. Our technical results concerning strong approximations and maximal inequalities are of potential independent interest.
About the Speaker:
Yingjie Feng is an assistant professor at the School of Economics and Management, Tsinghua University. He specializes in theoretical and applied econometrics, mathematical statistics and quantitative methods in social sciences, with particular interest in causal inference with panel data. Most of his work is motivated by empirical problems in applied economics and policy evaluation. He has developed novel semi-/non-parametric inference methods exhibiting robustness properties with respect to tuning parameter choices and other implementation choices.
Yingjie received his Ph.D. in Economics and M.A. in Statistics in 2019 from the University of Michigan. He also completed an M.A. in Economics in 2014 and a B.A. in Economics in 2011 at Peking University. Before joining Tsinghua University, he was a postdoctoral research associate at Princeton University.
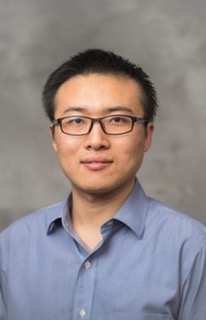
Your participation is warmly welcomed!
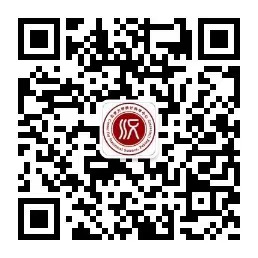
欢迎扫码关注北大统计科学中心公众号,了解更多讲座信息!