Principal flows, sub-manifolds and boundaries
报告人: Zhigang Yao (National University of Singapore)
时间:2023-05-11 15:00-16:00
地点:Room 1304, Sciences Building No. 1
Abstract:
While classical statistics has dealt with observations which are real numbers or elements of a real vector space, nowadays many statistical problems of high interest in the sciences deal with the analysis of data which consist of more complex objects, taking values in spaces which are naturally not (Euclidean) vector spaces but which still feature some geometric structure. I will discuss the problem of finding principal components to the multivariate datasets, that lie on an embedded nonlinear Riemannian manifold within the higher-dimensional space. The aim is to extend the geometric interpretation of PCA, while being able to capture the non-geodesic form of variation in the data. I will introduce the concept of a principal sub-manifold, a manifold passing through the center of the data, and at any point on the manifold extending in the direction of highest variation in the space spanned by the eigenvectors of the local tangent space PCA. We show the principal sub-manifold yields the usual principal components in Euclidean space. We illustrate how to find, use and interpret the principal sub-manifold, by which a principal boundary can be further defined for data sets on manifolds.
About the Speaker:
Zhigang Yao, 新加坡国立大学统计与数据科学系副教授兼终身教授,现为哈佛大学数学科学与应用中心成员,哈佛大学统计系访问教授,也曾作为特邀客座教授访问瑞士洛桑联邦理工大学(EPFL)等大学。Professor Yao的研究兴趣主要是复杂数据的统计推断,近年来专注于非欧式统计(Non-Euclidean Statistics)和低维流形学习。 近年来,Yao与其合作者提出在黎曼流形上重新定义传统PCA的principal flow/sub-manifold以及principal boundary等方法和理论,以及全空间下新的manifold learning方法和理论。这些方法通过考虑数据本身的非欧结构,旨在解决传统统计方法和理论中的缺陷。
个人网页:https://zhigang-yao.github.io/
Your participation is warmly welcomed!
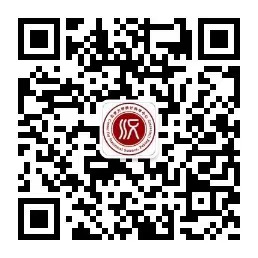
欢迎扫码关注北大统计科学中心公众号,了解更多讲座信息!