Variational Inference for Singular Models via Resolution of Singularities
报告人: Susan Wei (University of Melbourne)
时间:2023-05-24 14:00-15:00
地点:理科一号楼1365
Abstract:
Singular statistical models, ubiquitous in modern deep learning, lack identifiability and a positive definite Fisher information matrix. Motivated by a resurgence of interest in Bayesian deep learning, we set out to characterize the approximation gap in variational inference for the posterior distribution in singular models. The result rests on a central insight from singular learning theory according to which the posterior distribution over the parameters of a singular model, following an algebraic-geometrical transformation known as a resolution map, is asymptotically a mixture of so-called standard forms. We proceed to demonstrate that a generalized gamma mean-field family, following the resolution, can achieve a small approximation gap.
About the Speaker:
Dr Susan Wei is an assistant professor in the School of Mathematics and Statistics at the University of Melbourne. She is part of the Melbourne Deep Learning Group and a visiting faculty researcher at Google Research in Sydney. She currently holds a Discovery Early Career Researcher Award (DECRA) from the Australian Research Council (ARC). She received her PhD in Statistics from the University of North Carolina, Chapel Hill in 2014. Her research interests include statistics, machine learning, and deep learning.

Your participation is warmly welcomed!
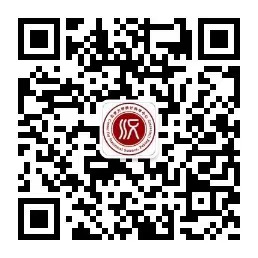
欢迎扫码关注北大统计科学中心公众号,了解更多讲座信息!