报告人: Philip Renyu Zhang(The Chinese University of Hong Kong)
时间:2022-09-29 15:10-17:00
地点:Tencent Meeting(280-219-727)
Abstract:
Large-scale online platforms launch thousands of randomized experiments (a.k.a. A/B tests) every day to iterate their business operations. Consequently, each user of the platform might be treated by a lot of A/B tests simultaneously. This triggers important questions of both academic and practical interests for a platform to best leverage the power of A/B tests: (a) How to estimate and infer the overall treatment effect of multiple experiments on the platform? (b) Without observing the outcomes of all experiment combinations, how to identify the optimal treatment combination? We leverage a novel framework that combines deep learning (DL) and double machine learning (DML) to estimate the heterogeneous treatment effect (HTE) of any treatment combination for each user on the platform. Our proposed neural network architecture combines interpretable and flexible structural layers. Our framework (called debiased deep learning, DeDL) exploits Neyman orthogonality and yields consistent and asymptotically normal estimators, thus allowing for valid inference of the treatment effects and best-arm identification. Furthermore, we collaborate with a large-scale video-sharing platform (Platform O) and implement our framework for 3 independent A/B tests on Platform O. Our DeDL approach outperforms the linear-regression- and deep-learning-based benchmarks to accurately estimate and infer the average treatment effects of any treatment combination, and to correctly identify the best-arm. Our statistical framework is further validated with synthetic data, which demonstrates its robust performance under model misspecification. From an application standpoint, this is the first study in the literature to validate the practical strength of the theoretically elegant DML method for causal inference by leveraging data from large-scale field experiments.
About the Speaker:
张任宇,香港中文大学商学院副教授(with tenure),快手经济学家&Tech Lead,主要研究数据科学方法论(包括数据驱动优化、机器学习和因果推断)及其在大规模在线平台运营策略的评估与优化中的应用。研究成果在Management Science, Operations Research, Manufacturing & Service Operations Management等顶级期刊发表并获得INFORMS, POM等多个学术共同体研究奖励。研究项目获得NSFC, SMEC, STCSM和HK RGC资助。担任学术期刊Production and Operations Management的Senior Editor和Naval Research Logistics的Associate Editor。在香港中文大学、纽约大学和快手内部讲授数据科学、运筹学和经济学课程。为快手平台开发经济学/数据科学方法论与框架,主要用于评估并优化平台宏观流量与营收生态(尤其是推荐系统和广告平台)。
个人网站:https://rphilipzhang.github.io/rphilipzhang/
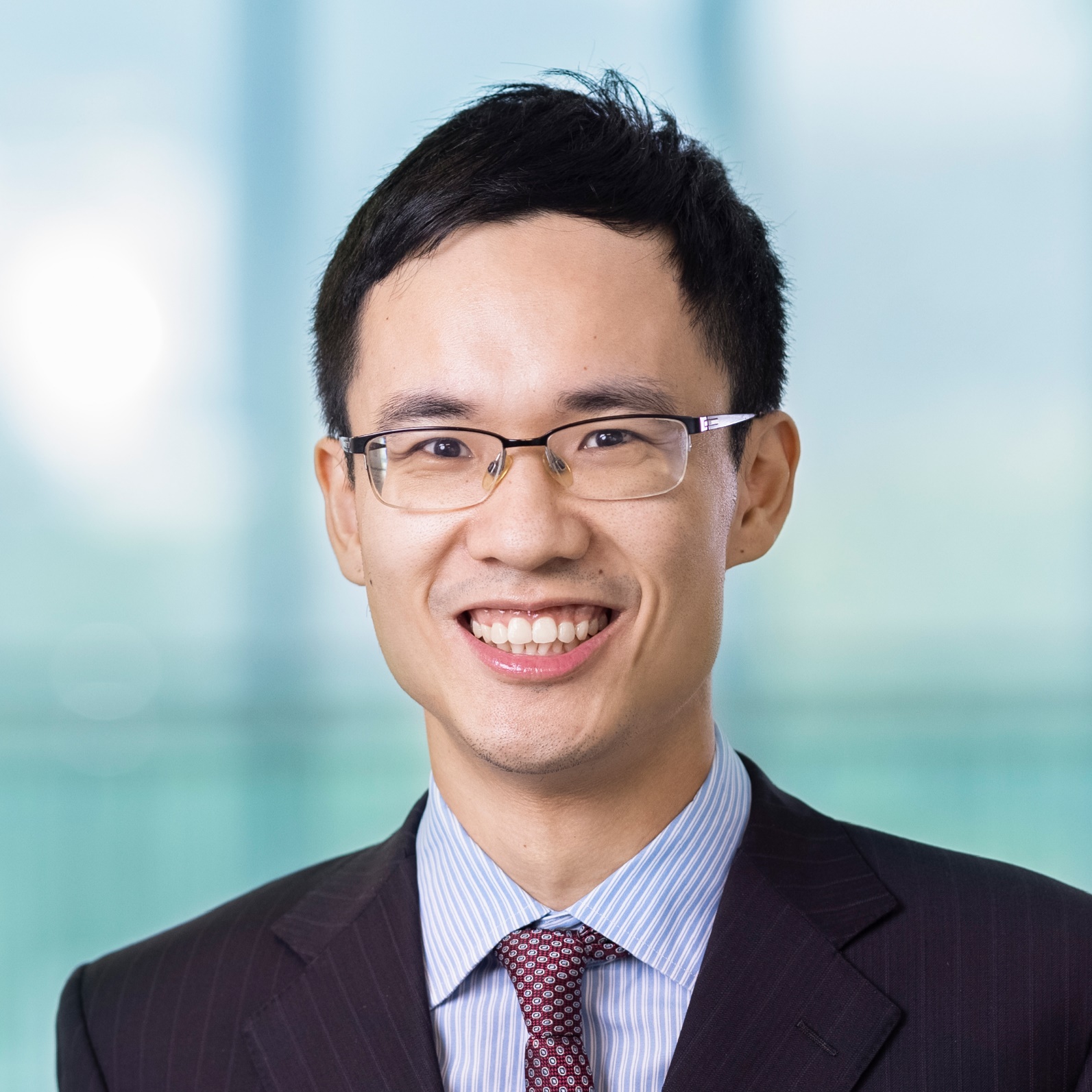
Tencent Meeting:https://meeting.tencent.com/dm/6zOpF39L7ZHS
Meeting ID:280-219-727
Your participation is warmly welcomed!
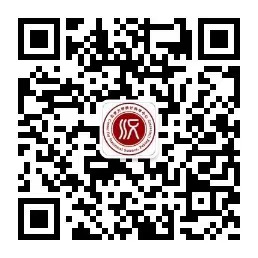
欢迎扫码关注北大统计科学中心公众号,了解更多讲座信息!