Parsimonious Model Averaging with a Diverging Number of Parameters
报告人: 张新雨
时间:2019-04-25 14:00-15:00
地点:光华2号楼217
Abstract:
Model averaging generally provides better predictions than model selection, but the existing model averaging methods cannot lead to parsimonious models. Parsimony is an especially important property when the number of parameters is large. To achieve a parsimonious model averaging coefficient estimator, we suggest a novel criterion for choosing weights. Asymptotic properties are derived in two practical scenarios: (i) one or more correct models exist in the candidate model
set; and (ii) all candidate models are misspecified. Under the former scenario, it is proved that our method can put the weight one to the smallest correct model and the resulting model averaging estimators of coefficients have many zeros and thus lead to a parsimonious model. The asymptotic distribution of the estimators is also provided. Under the latter scenario, prediction is mainly focused on and we prove that the proposed procedure is asymptotically optimal in the sense that its squared prediction loss and risk are asymptotically identical to those of the best – but infeasible – model averaging estimator. Numerical analysis shows the promise of the proposed procedure over existing model averaging and selection methods.
About the Speaker:
中科院系统所/预测中心研究员,主要从事模型平均和模型选择方面的研究工作,在统计学四大期刊和Journal of Econometrics发表论文十余篇,曾获三项自然科学基金委项目资助,目前担任《Statistical Analysis and Data Mining》、《系统科学与数学》和《应用概率统计》等期刊编委以及《Econometrics》客座主编。
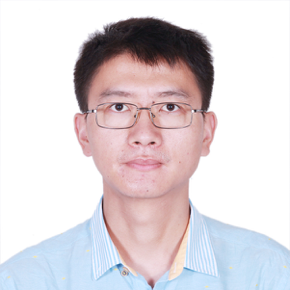