High-dimensional Tensor Regression Analysis
报告人: 张安如
时间:2019-06-20 14:00-15:00
地点:光华2号楼217
Abstract:
The past decade has seen a large body of work on high-dimensional tenors or multiway arrays that arise in numerous applications. In many of these settings, the tensor of interest is high-dimensional in that the ambient dimension is substantially larger than the sample size. Oftentimes, however, the tensor comes with natural low-rank or sparsity structure. How to exploit such structure of tensors poses new statistical and computational challenges.
In this talk, we develop a novel procedure for low-rank tensor regression, namely Importance Sketching Low-rank Estimation for Tensors (ISLET) to address these challenges. The central idea behind ISLET is what we call importance sketching, carefully designed sketches based on both the responses and the structures of the parameter of interest. We show that our estimating method is sharply minimax optimal in terms of the mean-squared error under low-rank Tucker assumptions. In addition, if a tensor is low-rank with group sparsity, our procedure also achieves minimax optimality. Further, we show through numerical study that ISLET achieves comparable mean-squared error performance to existing state-of-the-art methods whilst having substantial storage and run-time advantages. In particular, our procedure performs reliable tensor estimation with tensors of dimension p = O(10^8) and is 1 or 2 orders of magnitude faster than baseline methods.
About the Speaker:
Anru Zhang is an assistant professor at the Department of Statistics, University of Wisconsin-Madison. He is also affiliated to Machine Learning Group and Institute for Foundations of Data Science at UW-Madison. He obtained the PhD degree from University of Pennsylvania in 2015 and the bachelor’s degree from Peking University in 2010. His current research interests include High-dimensional Statistical Inference, Tensor Data Analysis, and Statistical Learning Theory. He received grants from the National Science Foundation and National Institute of Health.
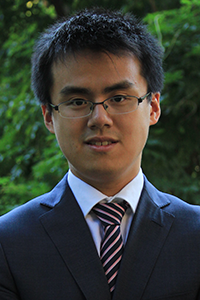