报告人: Stijn Vansteelandt, Ghent University
时间:2021-05-06 15:00-16:00
地点:Online(Zoom Meeting)
Abstract:
Inference for the parameters indexing generalised linear models is routinely based on the assumption that the model is correct and a priori specified. This is unsatisfactory because the chosen model is usually the result of a data-adaptive model selection process, which induces bias and excess uncertainty that is not usually acknowledged; moreover, the assumptions encoded in the resulting model rarely represent some a priori known, ground truth. Standard inferences may therefore lead to bias in effect estimates, and may moreover fail to give a pure reflection of the information that is contained in the data. Inspired by developments on assumption-free inference for so-called projection parameters, we here propose nonparametric definitions of main effect estimands and effect modification estimands. These reduce to standard main effect and effect modification parameters in generalised linear models when these models are correctly specified, but continue to capture the primary (conditional) association between two variables, or the degree to which two variables interact (in a statistical sense) in their effect on outcome, even when these models are misspecified. We achieve an assumption-lean inference for these estimands by deriving their influence curve under the nonparametric model and invoking flexible data-adaptive (e.g., machine learning) procedures. This talk aims to be broadly accessible, focussing on concepts more than technicalities.
About the Speaker:
Stijn Vansteelandt is an expert in statistical methodology for causal inference: a fast-growing field within statistics, which focuses on the development of statistical methods for inferring the causal effect of an exposure on an outcome from experimental and observational data under minimal and well-understood assumptions. He has authored over 175 peer-reviewed publications in international journals on a variety of topics in biostatistics, epidemiology and medicine, such as the analysis of longitudinal and clustered data, missing data, mediation and moderation/interaction, instrumental variables, family-based genetic association studies, analysis of outcome-dependent samples and phylogenetic inference. He has recently finished a term as Co-Editor of Biometrics, the leading flagship journal of the International Biometrics Society, and have previously served as Associate Editor for the journals Biometrics, Biostatistics, Epidemiology, Epidemiologic Methods and the Journal of Causal Inference. In 2020, he has joined the editorial board of the Journal of the Royal Statistical Society - Series B. His recent work focuses on strategies for obtaining valid inference for statistical and causal effect estimands when the analysis involves data-adaptive methods, such as variable selection or machine learning. Motivated by applications in personalised medicine, an additional strand of work focuses on causal prediction based on electronic health records: prediction of what the outcome for given individual would be if a given intervention were applied.
Zoom Meeting :https://zoom.com.cn/j/65329491531?pwd=dWsrU0E3cjhZb0ZNRUFCN0lNZllKdz09
Meeting ID:653 2949 1531
Password:830734
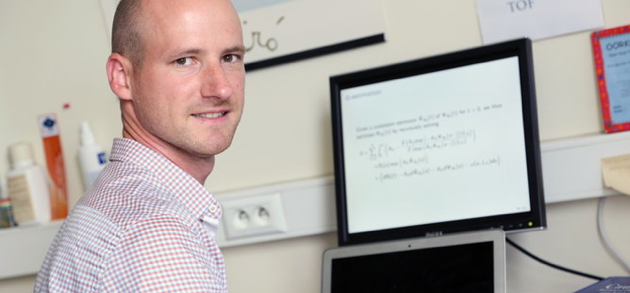
Your participation is warmly welcomed!

欢迎扫码关注北大统计科学中心公众号,了解更多讲座信息