报告人: Wei Ma(Renmin University of China)
时间:2021-12-15 10:30-12:00
地点:Tencent Meeting(979 3153 9451)
Abstract:
We consider the problem of estimating and inferring treatment effects in randomized experiments. In practice, stratified randomization, or more generally, covariate-adaptive randomization, is routinely used in the design stage to balance treatment allocations with respect to a few variables that are most relevant to the outcomes. Then, regression is performed in the analysis stage to adjust the remaining imbalances to yield more efficient treatment effect estimators. Building upon and unifying the recent results obtained for ordinary least squares adjusted estimators under covariate-adaptive randomization, this paper presents a general theory of regression adjustment that allows for arbitrary model misspecification and the presence of a large number of baseline covariates. We exemplify the theory on two Lasso-adjusted treatment effect estimators, both of which are optimal in their respective classes. In addition, nonparametric consistent variance estimators are proposed to facilitate valid inferences, which work irrespective of the specific randomization methods used. The robustness and improved efficiency of the proposed estimators are demonstrated through a simulation study and a clinical trial example.
About the Speaker:
马维,中国人民大学统计与大数据研究院副教授、博士生导师。研究兴趣包括自适应设计、临床试验设计与分析、生物统计、健康医疗大数据等。在Journal of the American Statistical Association、Biometrics、Statistics in Medicine、Statistical Methods in Medical Research等期刊上发表多篇学术论文。主持国家自然科学基金项目2项,参与国家重点研发计划1项。2018年至今担任中国现场统计研究会试验设计分会理事。
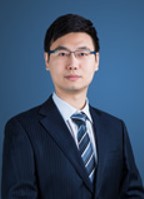
Tencent Meeting ID: 979 3153 9451
此讲座为北京大学公共卫生学院生物统计系、北京国际数学研究中心、北京大学统计科学中心联合学术讲座。
Your participation is warmly welcomed!

欢迎扫码关注北大统计科学中心公众号,了解更多讲座信息!