Abstract:
In this talk, we first propose a new class of metrics and show that under such metrics, the convergence of empirical measures in high dimensions is free of the curse of dimensionality, in contrast to Wasserstein distance. Proposed metrics originate from the maximum mean discrepancy, which we generalize by proposing criteria for test function spaces. Examples include RKHS, Barron space, and flow-induced function spaces. One application studies the construction of Nash equilibrium for the homogeneous n-player game by its mean-field limit (mean-field game). Another application is to show the ability to overcome curves of dimensionality of deep learning algorithms, for example, in solving Mckean-Vlasov forward-backward stochastic differential equations with general distribution dependence. The is joint work with Jiequn Han and Jihao Long.
About the Speaker:
Ruimeng Hu is an Assistant Professor at the University of California, Santa Barbara, with a joint appointment in Mathematics and Statistics and Applied Probability. Before that, she worked at Columbia University as a Term Assistant Professor. Her current research interests lie in the interdisciplinary area of machine learning, financial mathematics, and game theory. She is supported by an NSF grant and Faculty Career Development Award, Regents' Junior Faculty Fellowship at UCSB.
Zoom link: https://us02web.zoom.us/j/6817169181?pwd=bG5SWVE1Y0NWVzd6b3JjTEVEU1EyUT09
ID: 681 716 9181
Password: 123456
Your participation is warmly welcomed!
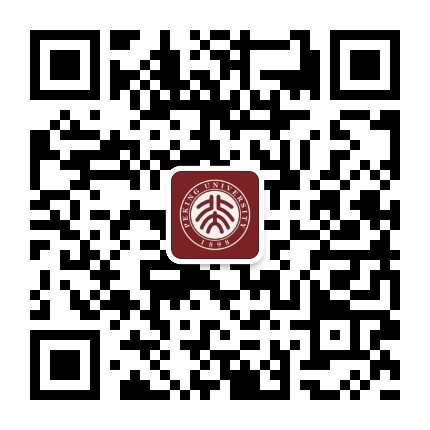
欢迎扫码关注北大统计科学中心公众号,了解更多讲座信息!