Holder: Yingxing Li (Xiamen University)
Time:2023-06-08 15:10-17:00
Location:Room 217, Guanghua Building 2
Abstract:
This paper proposes a novel large-dimensional positive definite covariance estimator for high-frequency data under a general factor model framework. We demonstrate an appealing connection between the proposed estimator and a weighted group-LASSO penalized least-squares estimator. The proposed estimator improves on traditional principal component analysis by allowing for weak factors, whose signal strengths are weak relative to idiosyncratic components. Despite the presence of microstructure noises and asynchronous trading, the proposed estimator achieves guarded positive definiteness without sacrificing the convergence rate. To make our method fully operational, we provide an extended simultaneous alternating direction method of multipliers algorithm to solve the resultant constrained convex minimization problem efficiently. Empirically, we study the monthly high-frequency covariance structure of the stock constituents of the S&P 500 index from 2008 to 2016, using all traded stocks from the NYSE, AMEX, and NASDAQ stock markets to construct the high-frequency Fama-French four and extended eleven economic factors. We further examine the out-of-sample performance of the proposed method through vast portfolio allocations, which deliver significantly reduced out-of-sample portfolio risk and enhanced Sharpe ratios. The success of our method justifies the usefulness of machine learning techniques in finance.
About the Speaker:
Yingxing Li is Professor of Statistics at Xiamen University. She received her Ph.D. from Department of Statistical Science at Cornell University. Her main research area is statistical estimation and inference for complex data. She has published papers in Annals of Statistics, Biometrika, Econometric Theory, Journal of Econometrics, Journal of the Royal Statistical Society (Series B) and etc.
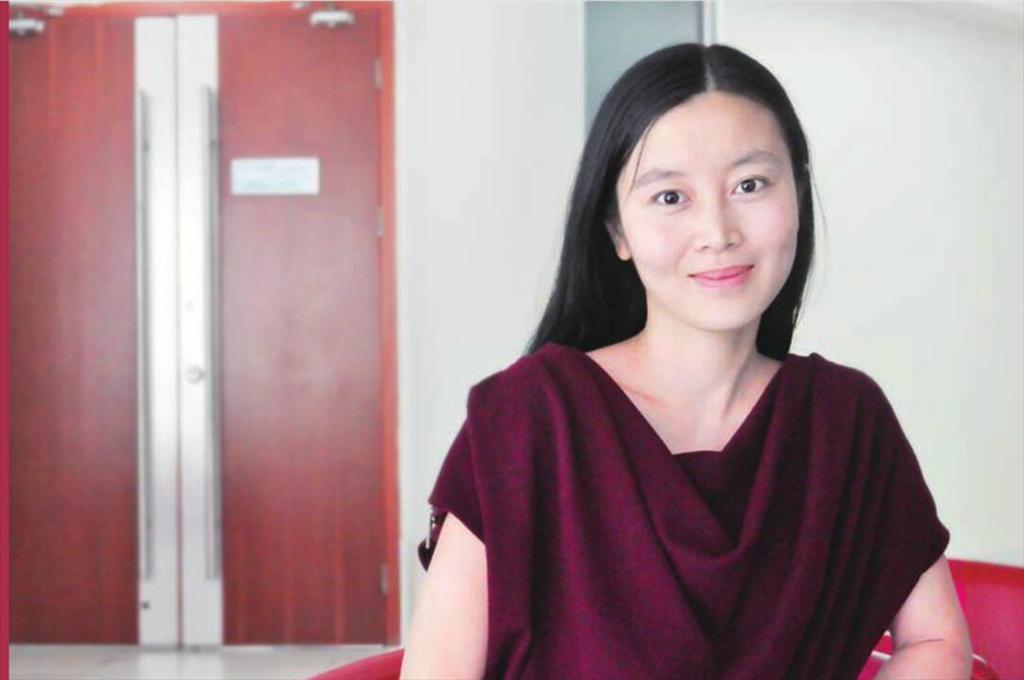
Your participation is warmly welcomed!
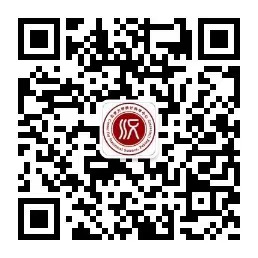
欢迎扫码关注北大统计科学中心公众号,了解更多讲座信息!