The Covariance Information is indeed Important for High-Dimensional Functional Data Modeling
Holder: Shaojun Guo (Renmin University)
Time:2023-03-30 15:10-17:00
Location:Room 217, Guanghua Building 2
Abstract:
The covariance function plays an important role in functional data analysis. In this talk we will present in two problems how to improve the estimation accuracy by utilizing the information of the covariance function. The first project considers estimating sparse covariance functions for high-dimensional functional data, where the number of random functions p is comparable to, or even larger than the sample size n. We propose the adaptive functional thresholding estimator and to handle the practical scenario where curves are partially observed with errors, we also develop a nonparametric smoothing version and its binned implementation to accelerate the computation. The second project presents a novel functional factor model for high-dimensional functional time series that maintains and makes use of the functional and dynamic structure to achieve great dimension reduction and find the latent factor structure. To estimate the number of functional factors and the factor loadings, we propose a fully functional estimation procedure based on an eigenanalysis for a nonnegative definite matrix which is a combination of covariance functions. Asymptotic properties of the proposed method are studied when p diverges at some polynomial rate as n increases.
About the Speaker:
郭绍俊,现为中国人民大学统计与大数据研究院长聘副教授。2003年本科毕业于山东师范大学,2008年获得中国科学院数学与系统科学研究院理学博士学位。2008年-2016年任中国科学院数学与系统科学研究院助理研究员。2009年-2010年赴美国普林斯顿大学运筹与金融工程系博士后研究,主要研究方向为高维数据分析。2014-2016年赴英国伦敦经济学院统计系做博士后研究,主要研究大维时间序列建模。目前主要研究方向有:统计学习;非参数及半参数统计建模;生存分析及函数型数据分析等。研究成果发表于Journal of Royal Statistical Society Series B, Journal of the American Statistical Association, Biometrika, Bernoulli, Journal of Econometrics, Journal of Business and Economic Statistics等期刊上。
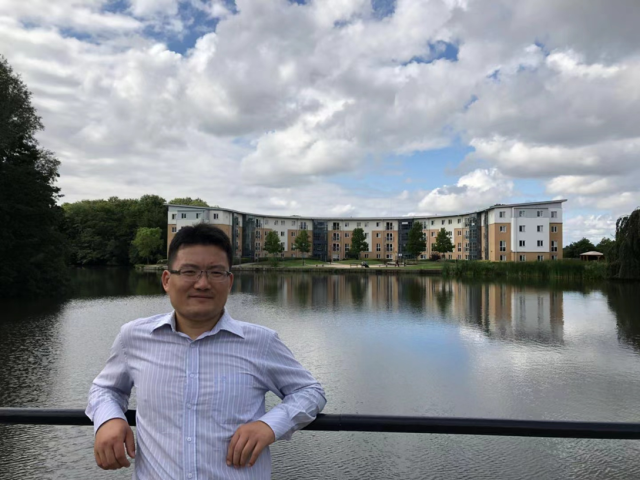
Your participation is warmly welcomed!
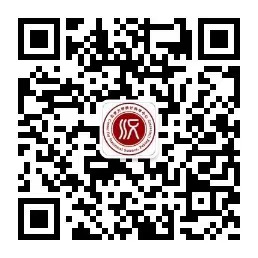
欢迎扫码关注北大统计科学中心公众号,了解更多讲座信息!